Share
Security Research
Proof of Quality
September 21, 2023
•
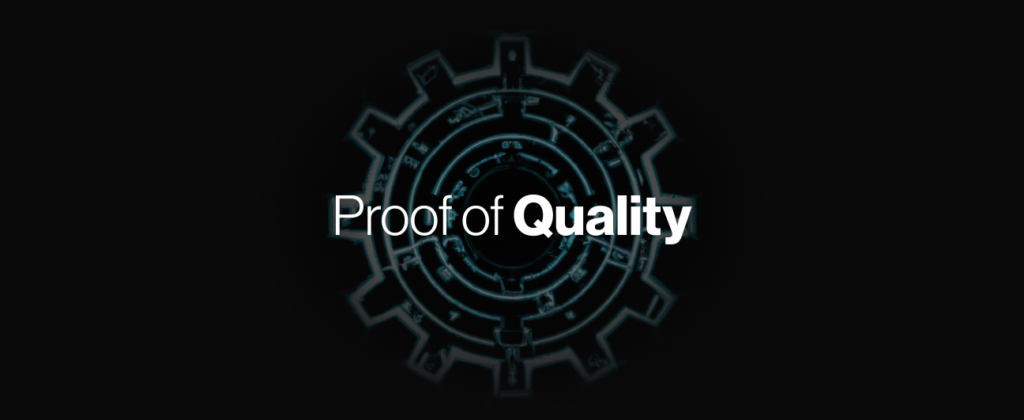
Forta is a real-time detection network for security monitoring of blockchain activity. The decentralized Forta Network scans all transactions and block-by-block state changes, leveraging machine learning to detect threats and anomalies on wallets, DeFi, NFTs, bridges, governance and other Web3 systems. When issues are detected, Web3 infrastructure can respond to prevent attacks via transaction screening and incident response.
In the ever-evolving landscape of cybersecurity, making informed decisions about security solutions is vital. While it’s easy to get swayed by buzzwords and high-level statistics, consumers need a transparent and objective way to evaluate the quality of security solutions. The proposed ‘proof-of-quality’ is to provide that clarity in the Web3 space.
In traditional Web2 environments, third-party evaluations by SELabs, MITRE, etc. adopt a black box testing approach (primarily due to privacy and proprietary concerns). They create a variety of test scenarios - both synthetic as well as real-world samples - to assess a set of solutions side-by-side. In contrast, the Web3 ecosystem — of which Forta, as a crypto native, open and permissionless protocol, is an integral part — provides an opportunity for a different approach. Forta's detection bots can provide a ‘proof-of-quality’ through radical transparency.
Key Metrics and Methodology
Before diving into the specifics of 'proof-of-quality,' let’s first understand the crucial metrics and methodological considerations that define the quality of a security solution.
Transparency with Key Metrics:
Proof-of-quality necessitates disclosure of key metrics and raw data utilized to calculate the metrics. These are:
1. Recall: The term ‘recall’ measures the system's ability to identify threats. It's the percentage of total threats the system can identify. Ideally, you’d want this number to be as close to 100% as possible. If a system fails to identify a threat, it’s termed as a False Negative (FN).
2. Precision: Precision, on the other hand, ensures that the system only flags real threats. It is calculated as the percentage of identified threats that were genuinely hazardous. Mistakenly identified threats are termed False Positives (FPs), and they can be troublesome, causing alert fatigue or user friction.
It's crucial to understand that there's often a tradeoff between precision and recall. A system could theoretically achieve 100% recall by flagging everything but would suffer in precision. Conversely, a system with high precision but low recall might miss out on many actual threats.
Comprehensiveness
These two metrics, however, are not sufficient. A comprehensive set of metrics need to be disclosed.
The impact of False Positives and False Negatives varies. For instance, wrongly flagging a popular smart contract as malicious will have more significant consequences than doing the same for a less popular one. Weighted metrics can account for such differences.
A singular percentage number (like 75% precision) is insufficient without context. Confidence intervals provide a range within which a repeated measurement would fall a certain percentage of the time (e.g., 90%).
Methodology Disclosure
Lastly, there are methodological considerations. How are the measurements taken? Are they guided by expert evaluations or crowd-sourced? How is the data sourced and sampled and are the potential biases being captured? Understanding the methodology is crucial to understand how metrics are derived, empowering everybody to derive the same metrics themselves if they so choose.
Scam Detector Proof-of-Quality
The Scam Detector adopts proof-of-quality and aims for radical transparency revealing it’s quality of such that anybody can understand, assess and reproduce these metrics.
Transparency: All metrics are publicly disclosed in the quality_metrics.json within the Scam Detector GitHub repository. The raw data can be requested here at any time; in the coming weeks, the data will be made available directly.
Comprehensiveness: Metrics like precision, weighted precision, recall, and weighted recall are calculated. The Wilson Score Interval is used for confidence intervals at a target level confidence of 90%.
Methodology Disclosure: Precision metrics are evaluated by trained experts within the Forta Community, adhering to documented grading guidelines. Recall metrics are sourced from publicly disclosed scams, primarily from Twitter, acknowledging a potential bias toward more prominent scams.
Future Directions and Community Involvement
The proposed 'proof-of-quality' is a step toward greater transparency and more informed choices in Web3 security solutions. It's a start but certainly not the finish line. It requires community involvement for refinement and enhancement.
For feedback or to shape this approach further, please contact us at community@forta.org and/or join the Forta Threat Research Initiative.
Opting for radical transparency sets a standard that benefits consumers and the industry alike. Your input will make it even more robust. Thank you for being a part of this journey toward a safer, more secure Web3 ecosystem.
Subscribe to Forta’s News
Stay updated on the latest Forta news and announcements.